Situational awareness
Based on the Cynefin framework
This contains substantial material from Cynefin Training programmes and Dave Snowden's blog posts. The author acknowledges that use, and further that his use of that material are his own and should not be considered as being endorsed by with theCynefinCompany or Dave Snowden.
Knowledge and perception
Cynefin classifies the objective reality into three types of system - Order, Complex, Chaotic. We also saw how to distinguish between the types of system using the concept of constraint. Those three types of system are objective and they can be used to describe and understand the natural world.
However, human knowledge is intrinsically a subjective construction, not an objective reflection of reality. Human perception is important for action and Cynefin is an action based framework. This means that the emphasis should shift from the apparently objective systems around us to the cognitive and social processes by which we construct our subjective models of those systems.
This constitutes a major break with traditional systems theory, which implicitly assumed that there is an objective structure or organisation in the systems we investigate. This is reinforced by the concepts of autonomy and self-organisation which imply that the structure of a system is not given, but developed by the system itself, as a means to survive and adapt to a complex and changing environment[1].
That's why Cynefin also has four states (Clear, Complicated, Complex, Chaos) that present the ways we we know things and accordingly we act. Each is characterised by an appropriate decision model. Cynefin divides Order into two domains - Clear and Complicated. Because with human beings we are dealing with phenomenology and epistemology (human perception and human knowledge are important part of the system) the line between Clear and Complicated is a phenomenological boundary and not an ontological boundary. The other boundaries are ontological. In Clear domain the constraints are rigid.
Most importantly, the Cynefin framework is not about “objective” reality but about perception and understanding. We understandi that different people might be perceiving and thus hold different perspectives on the same event or situation. Such an understanding can be used to one's advantage. What we care most about is how people perceive and make sense of situations in order to make decisions. Accordingly in Cynefin we five domains - Clear, Complicated, Complex, Chaos, Confused, that help us understand how humans perceive the situation they are in [2].
In summary Cynefin has three ontological states (Order, Complex, Chaotic), four epistemological states (Clear, Complicated, Complex, Chaos), and five phenomenological domains (Clear, Complicated, Complex, Chaos, Confused) [1].
Some people see the differences between Order, Complex, Chaotic as only about perception. In Cynefin we see the differences as being about reality, knowledge and perception - hence the three types of system and five domains.
In order to get better alignment inside an organization what we actually need is to increase the friction between perception, knowledge and reality. If we allow reality, knowledge and perception to separate wildly everything becomes totally inauthentic. If we increase the interaction (friction) between perception, knowledge and reality they will never be fully aligned but at the same time the misalignment never gets too far.
Cynefin Domains
The five Cynefin domains are presented graphically as the Cynefin model. These are the five main situations in which human beings would make decisions. The focus of a domain name is on the nature of the system it represents. The action phrases (e.g. probe-sense-respond) reference how we should gain knowledge and act. Methods and practices also have characteristics that place them in domains or in transition between domains. Hence the designation of Best/Good/Exaptive/Novel practice.
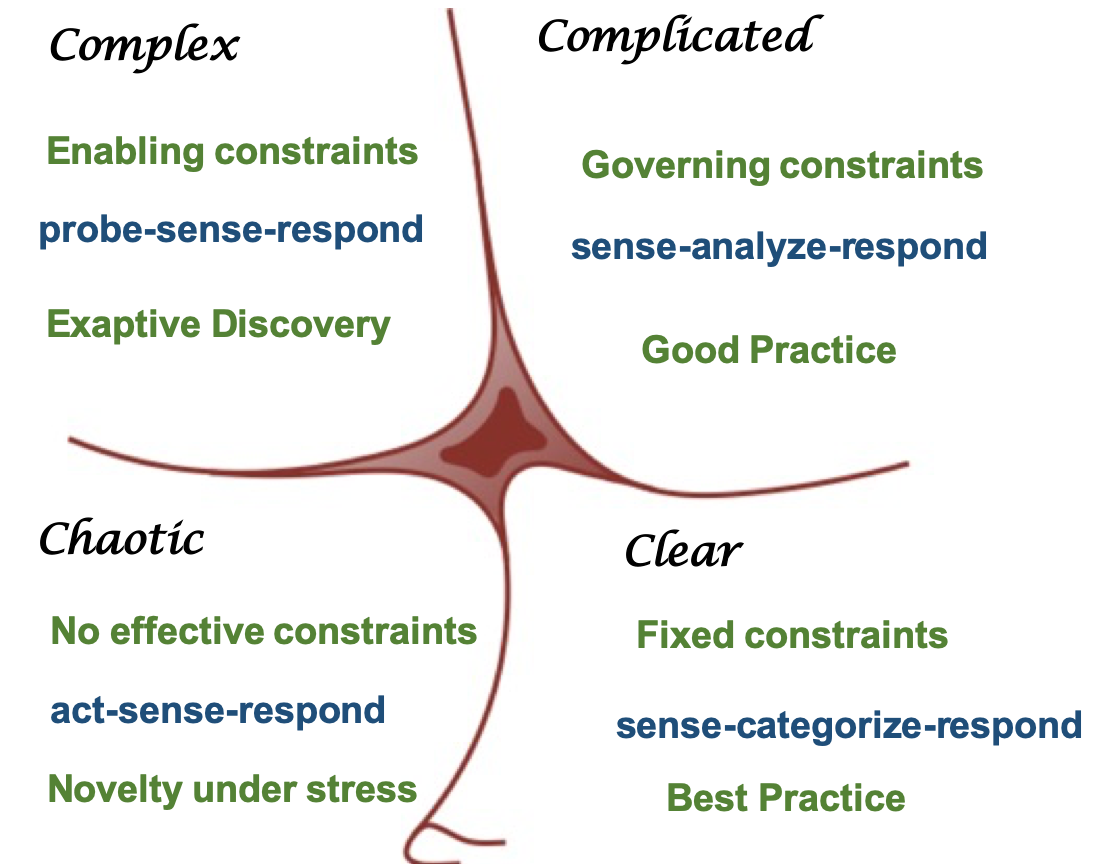
The right-hand domains (Complicated, Clear) are those of order, and the left-hand domains (Complex, Chaos) are those of un-order. Un-order is not the lack of order, but a different kind of order and is legitimate in its own way[1]. The central area, is the domain of Confusion. The shift between order and chaos is shown as a catastrophic fold - a walk over the cliff without realising what will happen until it is too late. It is to show how easily obvious solutions can cause complacency and fall into chaos.
Cynefin divides Order into two domains - Clear and Complicated. The separation is a question of perception - some see an issue as obvious while others see it as hopelessly complicated. We may say that Clear is visibly ordered and Complicated is ordered too but hidden so that we have to discover it. The separation is not “objective”, but about human perception and knowledge. As we saw earlier the boundaries between the other three domains are about objective reality, knowledge and perception all together.
Each domain has its own particular relationship between cause and effect. With Cynefin we can avoid using a practice established on assumptions of causality in the non-causal domains and vice versa. In the ordered domains there is a known, obvious and self evident (Clear) or knowable (Complicated) relationship between cause and effect, In the complex domain there probably isn't a relationship between cause and effect and even if there is it is nonlinear, and delayed. Such a cause and effect relationship is called retrospective coherence. It means that, in hindsight, it's easy to explain why things happened, yet it is impossible to predict them ahead of time. Hence it cannot be used to act in the present. Some caution is in order - retrospective coherence may be perceived as ”self evident”! In chaos there is no relationship between cause and effect at all - the system is in a sense random.
Cynefin is a fractal framework. Fractality means we may find something to be complicated on one level but complex on another. Organisation faces situations, issues and such that can be placed within the various Cynefin domains. The organization as a whole can be in multiple domains or - and this is critical, in a state of dynamic shift between domains.
All four domains have equal value. We need to really emphasize this: the essence of the Cynefin approach is not to say complexity is good, order is bad. There is nothing wrong with order if we can maintain constraints because it produces predictability.
The key is to understand in which domain we are in and as importantly how we plan to move between them.
Clear domain
In the Clear domain the constraints are rigid and fixed. Cause and effect relationships are known, generally linear, empirical in nature, and not open to dispute[1]. Linear relationship between cause and effect not only exists but its nature and consequential actions are self-evident to any reasonable person. For example. driving on the same side of the road - left side in Britain and right side everywhere else. That's the reasonable thing to do because everybody does the same.
In the Clear domain the way we know things and act is: sense, then categorize and respond based on best practices.
Clear problems have known solutions. Since constraints are fixed no deviation is allowed and organizations employ best practice to manage their processes. Best practices incorporate solutions to particular problems, stored as recurring patterns allowing for high performance as they comprise of detailed and precise steps, sometimes at the edge to automatic responses. They minimize costs, reduce uncertainty and increase control over processes In this perspective deviations from rational decisions are labelled as “biases and errors” primarily caused by human error e.g. "operator error, organisation error, maintenance error, design error, installation error and assembly error”. Applying a best practice doesn't need experts - what to do is clear to everyone. However, defining appropriate best practices is primarily reserved to the organisation's experts.
Organisational structures are bureaucratic, processes are oriented towards formal procedures and controls. Structured techniques are not only desirable but mandatory. As the future is believed to be predictable and full information is available, decision makers will stick to single-point forecasting. Single-point forecasting is when we assigne a single value to an outcome e.g. to forecast that the temperature at a given place on a given day will be 23 degrees Celsius. In contrast probabilistic forecasts assign a probability to each of a number of possible outcomes.
In clear situations, the focus is on efficiency and consistency[1]. We monitor for deviance but for two different purposes. The first is focused on compliance, the latter on checking if its time to change. In the Clear domain predictability here is both a benefit and a danger[2].
In the Clear domain KPIs work, are valuable and necessary, but they need to be easy to understand, few in number and not require constant reference to manuals or half remembered training[2].
Complicated domain
In the Complicated domain the constraints are only governing, but within them we've got predictability. The difference between rigid constraints (Clear) and governing constraints (Complicated) is important. In the Complicated domain the right approach is not clear and self-evident because there are more than one credible alternative solutions. Hence we have to deploy expertise to select one of the alternatives.
In the Complicated domain the way we know things and act is: sense, then analyse and respond.
Complicated problems, even with many thousands of components, have a few predictable solutions. It is believed that he environment and organisations can be fully described, even if that might not be easy or obvious at first glance. We can prepare and use models including systems of differential equations and other high level math. As cause and effect relationships are knowable and supposed to be given by "nature" or any other external authority experts will in turn favour experiments, fact-finding and scenario-planning[1] While stable cause and effect relationships exist in this domain, they may not be fully known, or they may be known only by a limited group of people[1]. Within the governing constraints variation is allowed. In this knowable domain, experiment, expert opinion, fact-finding, and scenario-planning are appropriate[1]. If we've got the right expertise and the right training and it is acknowledged by our peers then a degree of variation is permitted. A methaphor could be the improvisation in jazz - one governing theme and variation based on individuals.
With governing constraints we have good practice and not best practice. The difference between best and good practice is important. A best practice doesn't need experts - it is obvious. if you have to think about it, we can agree on a good practice. Some caution in order: if we impose best practice in a good practice domain the people that understand the practice realize there are variations but they are not allowed to perform them anymore so they will drive things underground.
In the Complicated domain the way we know things and act is: sense, then analyze and respond based on good practices.
The organisational structures are oriented towards bureaucracy and technical determined processes, managed by formal procedures. Controls are relaxed to allow for variations based on expertise: The right to vary practice is hard won and to a degree bounded by peer review and peer acceptance. Planning and control can be far less explicit because the basics of what are needed are embedded in the professional standards, practices and training. In this domain alignment on goals and common purpose and goals are useful as they act as a constraint on deviant behaviour. Goals and targets should not be too many and should not be created by bureaucrats or consultants who lack experience of the context, membership of the profession and peer acceptance.
As an example we can look at how an expert statistician is deciding on what of the many statistical tests to use for inference:. The key for a successful analysis of an experiment is to adopt suitable statistical tests. It is crucial to understand the relationships among the statistical methods and to properly integrate the individual methods to effectively apply them. The choice for valid statistical tests greatly depends on the dependency of the sample and the number of independent variables in the analyses as well as the measurement scale of dependent variables and independent variables. A decision tree starting with major research questions appears in Fig 2[14].
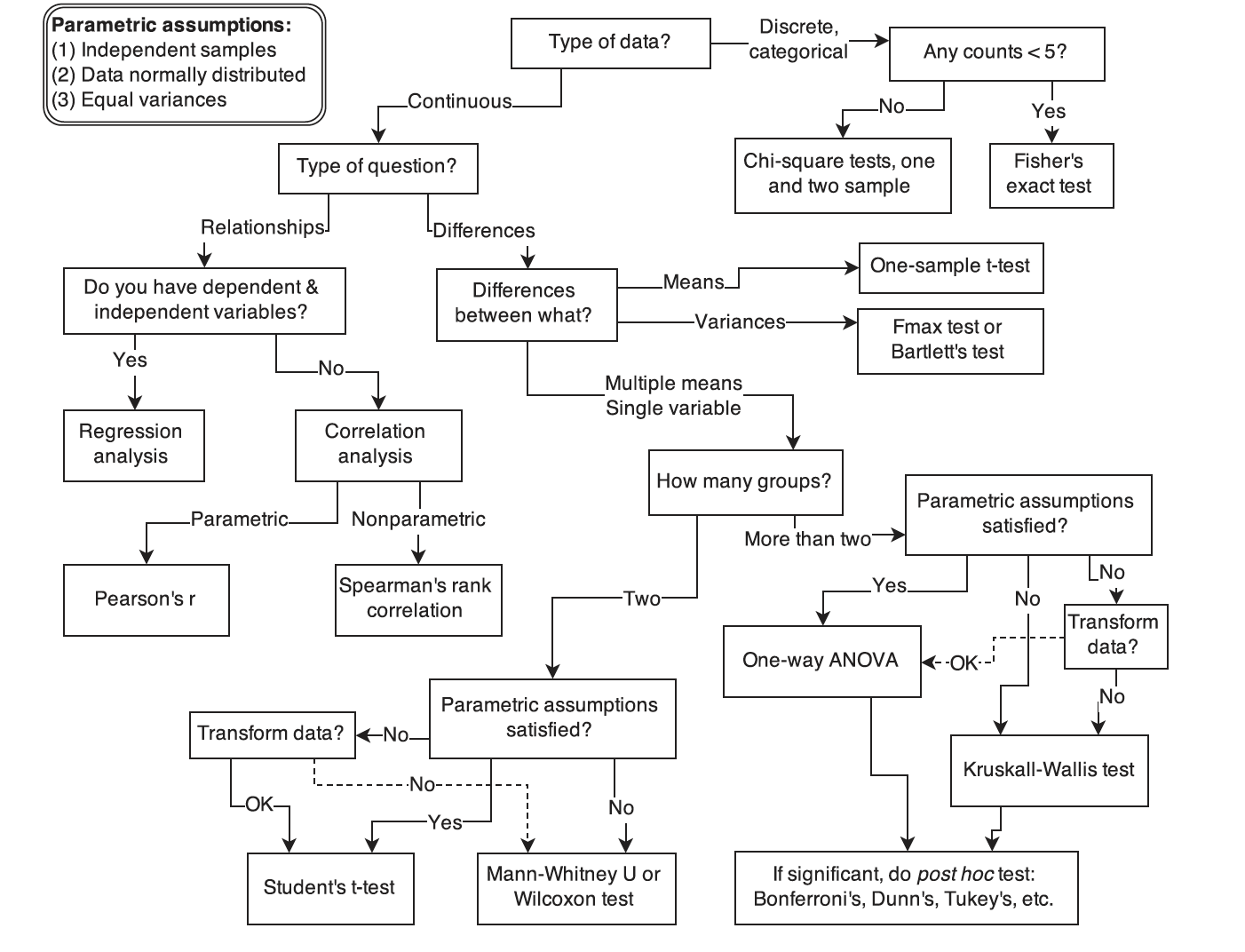
Choice among techniques depends on how the expert would answer each of the "Yes/No" questions.
Decision makers have to rely on expert opinion. Experts here are understood as professional individuals who, through defined training programmes, acquire a specialist terminology; codified in textbooks. The high level of abstraction is teachable given the necessary time, intelligence and opportunity. As decision makers are regularly not proficient enough in the respective field, relying on expertise shifts authority away from formal hierarchical positions to rather closed communities differentiated from the rest of the organisation by a professional identity and a specific language.
The problem in this domain is to stay open for changes despite of experts and trivialisation, thus, "management of this space requires the cyclical disruption of perceived wisdom”[3]
Complex domain
In the Complex domain there are constraints but they are very loose. This might be perceived as if everything is connected to everything else but in mysterious ways. We call them enabling constraints because they nudge the system to move in a direction..
The difference between a governing constraint and an enabling constraint is like the difference between an endoskeleton and exoskeleton. For an insect with an exoskeleton everything is contained within the skeleton and doesn't allow for much variation - that's a governing constraint. With an endoskeleton we've got coherence but it allows for massive variation - that's an enabling constraint [4].
In the Complex domain there are cause and effect relationships but both the number of components (agents) and the number of relationships defy categorisation or analysis. In complex environments recursive effects render impossible the rational choice of actions, grounded in causality. Different observers will end up with different explanations for the same observation. In a complex system every intervention changes the thing observed. We have inherent uncertainty. The agents and the system coevolve. Yet, system behaviour is not chaotic or random. Order emerges from interactions of the agents and the system over time. The form of that order is unique to each emergence as it is path dependent. History is important as different starting conditions give different outcomes so the system is irreversible. Emerging patterns can be perceived but not predicted - we call this phenomenon retrospective coherence [3]. We can't rely on any retrospective e.g. lessons learned, process because if the result was positive or not determines how people would describe what happened.
One thing that we can say with absolute confidence about complex systems - whatever we do will produce unintended consequences. That's the absolutely guaranteed certainty. So whatever we do something would happen which we didn't expect or didn't planned for. That means we are responsible for the unintended consequences. Even though we couldn't have predicted the individual outcome we knew there would be unintended consequences so we should have planned for it. The argument from that is that we need to do small things early so that the unintended consequences are more manageable.
How do we know we are in a Complex domain? The basic heuristics are - if the evidence supports competing hypothesis, if there is a dispute, if time pressure is high assume it's complex. The general default is: if in doubt assume it's complex. What if for the expert, it is Complicated and for the rest it's Complex? That's OK. If we trust the expert, we can treat is as Complicated even though it is Complex for the rest. If we don't trust the expert, then we have to treat it as Complex. We can accept that something is complicated without understanding it - that's the essence of Humanity. We have to be able to trust people to make decisions for us.
The decision model is: probe via experiments, sense the results and respond by either amplifying of good or dampening of bad emerging patterns [5].
We can safely claim that human systems are complex by default. Why? Because they are comprised of humans. Humans are not consistent in their behaviour. The most important indicator of how we woud act, is the context. The situation or place we find ourselves may completely change our perception and judgement. Modeling of human systems is possible. As Murray Gell-Mann, who received the 1969 Nobel Prize in physics famously said: “The only valid model of a human system is the system itself”[6].
Here is an bit more subtle example of that - the “shadow or informal organisation. That complex network of obligations, experiences and mutual commitments grounded in shared experiences, values and beliefs without which an organisation could not survive[3]. To convey knowledge informal networks prefer stories and proverbs. As such a language is difficult to comprehend and use for outsiders, these networks tend to be closed and cohesive circles, more rigidly restricted than professional one, and rather excluding than including experts. Membership, even if embedded in formal organisations, is often informally regulated and based on emotions, common experience, values or beliefs, with a long time of apprenticeship, (transition) rituals and enforcing myth form stories, and long tenure[7]
Different types of problems require different management approaches.
The nature of the system determines the way we acquire knowledge about it and manage it. Chaotic and ordered systems can be managed in a structured way. With complex relationships in this domain, relying on expert opinions based on historically stable patterns of meaning will insufficiently prepare us to recognise and act upon unexpected patterns”[1]
For complex problems the role of management is to create and relax constraints as needed and then dynamically allocate resources where patterns of success emerge. We manage what we could manage - the boundary conditions, the safe-to-fail experiments, rapid amplification and dampening. By applying this process, we discover value at points along the way.
Managing complex systems is changing the present without of a series of stepping stones but with a sense of a rough direction with willingness to change it [8].
In a complex system we can only manage the evolutionary potential of the present. We can't plan long term, because the cause ond effect relationships will not be fully known or knowable in advance, but understood only retrospectively in hindsight. Long term planning is at best a sense of direction rather than a set of goals and targets. A sense of direction can be achieved by visionary goals, but their use has to be with care. We can plan very short term while staying alert and exploiting patterns of possibilities as they start to become visible. However it is not just about responding to patterns, we can manage the constraints, the catalysts of patterns and the allocation of resources. It is about creating the conditions in which radical re-purposing is easier to stimulate and easy to recognise. Rapid feedback loops, fast failure and learning, experimentation are all key approaches. The plans and the controls will emerge as we do that. Accordingly we can only really measure direction and speed of travel from the present.
Exaptation is when under condition of stress a trade developed for one function leaps sideways, is re-purposed for a different function/capability altogether [9]. If we look at the evolution - re-purposing happens when the system is under stress. If we stress any life form mutation rate increases fast. That produces more casualties but also more variation and more exaptive capacity. Adaptation is a linear evolutionary process - each change happens gradually over time. So in evolution we have a linear adaptive process, then we get a stress based natural leap and then another linear process. Exaptation accounts for the speed of the evolution. The key on exaptation is to repurpose things in which you are already competent, so the learning or energy needs are reduced[10]. Moving to higher levels of abstraction allows us to combine and recombine things in novel ways.
Enabling constraints allow for huge degree of variety but there is still governance within the system. If we manage to encapsulate the enabling constraints in the form of governing heuristics or better still in the form of habits, then we've got a governing structure we can rely on [11].
In human systems the enabling constraints are called governing heuristics. Generally, decision makers in complex contexts rely for a substantial proportion of decisions on heuristics that are adapted to their specific environment. In the Complicated domain we have rules, in Complex we have governing heuristics. Governing heuristics are one of the ways to embody expert knowledge. Contrary to rules designed to include specific solutions for specific problems, heuristics provide a common structure for a range of similar problems, but also allow for the necessary flexibility in turbulent complex situations and unexpected events. Heuristics handle ambiguity whereas the rules don't.
Examples of governing heuristics are “Satisfy the customer through early and continuous delivery of valuable software”, “Welcome changing requirements, even late in development.”, “Deliver working software frequently”. Actually all twelve principles from the Agile Manifesto are governing heuristics i.e. enabling constraints. Another example heuristic comes from the US Marine Corps - if the battlefield breaks down: capture the high ground, stay in touch, keep moving [11]. Notice that heuristics are measurable for compliance. They are not statements of general principles; those aren't heuristics in this sense because they are too ambiguous. They are measurable - did you capture the high ground, did you stay in touch, did you keep moving? In a governing heuristic compliance must be measurable without ambiguity. Applying broad principles like “Don't be evil!” won't work.
Actually any strategy is an enabling constraint. It gives an organization a direction. Without a direction there is chaos.
We know that if we follow the heuristics there will be a degree of failure but that's acceptable because it's considered inevitable. If we try to force a non-failure culture in a complex system, things will get nasty fast.
Chaos domain
In Chaos domain there are no constraints. Searching for right answers is pointless, because the relationships between cause and effect are impossible to determine. There is a potential of order but few can see it and if they can they rarely do unless they have the courage to act. In this domain, our immediate job is not to discover patterns but to act.
Making something chaotic is very difficult to do. If we go into chaos deliberately it takes a huge amount of energy to create it. It is rather like nuclear fusion - the energy required for the magnets to contain the plasma is more than the energy we get out of the nuclear reaction.
The decision model is to act quickly and decisively, then sense and respond.
Chaos is the domain of crisises and novel practices. In a crisis it's all about control and little about planning. There is no time to be nice or consultative so the imposition of control is likely to be draconian in nature. The real value comes in working out what to impose and its shouldn’t be a final solution; the essence of good crisis management is to create enough constraints that you shift the problem into complexity which means some patterns are starting to emerge[snowden_liminal_2019a].
In the Chaotic domain novelty arises from stress, from a complete break with the familiar. As a result radically different approaches would be accepted while in normal times they won't. In order to be effective the new ways must be accepted by all reasonable people. The case in the chaotic domain is that the context makes unreasonable stubbornness more difficult to sustain[12]. Chaos is relative to the context. We can get micro-chaos as well in a specific context which is in general constrained. As far as the context is concerned we may have no relationships between cause and effect and all of a sudden someday somebody makes a novel connection.
For example. the invention of the microwave ovens. In 1943, an engineer, Percy Spencer was building a magnetron when he noticed a chocolate bar melt in his pocket. He realised the connection and placed an egg in a kettle and directed the microwaves from the magnetron into a hole cut in the side of a kettle. The egg exploded and we had the first microwave. Raytheon filed the patent in 1945 and made a fortune [13].
Before going to the Confused domain, let's remind ourselves about that catastrophic fall from Order into Chaos. It happens when we make the system very constrained. Sooner or later the tensions build up in the system and there is a disaster. Breaking the laws becomes a norm, corruption follows, everything else follows through.
Confused domain
The Confused domain is in the middle of the Cynefin diagram. This domain is as significant as the others in that it is an entry point into the sense-making process. Remember Cynefin is a decision support framework so its focus is on understanding the underly nature (ontology) of the problem being addressed [12].
This domain is associated with the idea of perplexity. It is a state of not knowing which domain we are in. There are an authentic and inauthentic ways to deal with such a confusion.
The authentic way is to acknowledge upfront that we are in a confused state and then seek to explore options to exit. It's authentic to use confusion as a transitionary device and eventually understand in what domain the present situation is in. Thus when confused we are at an entry point into the sense-making process. In the Confused domain we have primary sense-making, namely creating sufficient structure of constraints to shift into a domain where the nature of action is known, We can get into authentic confusion when we deliberately create a state of ambiguity or stress in order to achieve a transition. Some people call such a state “aporetic” or "perplexed". The Oxford English Dictionary defines aporetic as a state of “perplexity or difficulty”. Aporetic means in a state of aporia. In philosophy, an aporia is a puzzle or a seemingly insoluble impasse in an inquiry, often arising as a result of equally plausible yet inconsistent premises (i.e. a paradox). In Plato's Meno dialogue Socrates describes the purgative effect of reducing someone to aporia: it shows someone who merely thought they knew something that they do not in fact know it and instills in them a desire to investigate it.
The inauthentic way is not to acknowledge we are confused or acknowledge it but unable to come out of it. Staying in confusion is a bad place to be in. In inauthentic confusion we assume we are in the domain in which we feel most comfortable. We feel certainty when uncertainty should be the norm. It is a state of false confidence, assuming we know how to act without first making anontological situational assessment. An example of inauthentic state is when trying to apply Six Sigma practices in software development projects. That is using Complicated techniques while in a Complex situation.
Human beings situational assessment is often done on the basis of an action they have already decided to do. In other words - we assess a situation having already decided how to act. In general humans try to apply one universal approach in a situation where different contexts require different solutions to be applied.
That is what traditional management consultancies usually do. Instead of trying to discover the ontological context of their clients they come with an ontological assumption that it is ordered. As we know in the complicated domain we need good practices and in the clear domain we need best practices. Traditional management consultancies embody good and best practices in business cases. However, the case-based approach is very dependent on understanding of the context from which the originating cases were derived. If we try to repeat what worked before, the context may have shifted. We probably won't fully understand the context anyway therefore we can't rely on it to the same extent. The fundamental danger with the case based approach is the confusion of correlation with causation. The fact we've got a correlation doesn't mean we've got a causal link. Trying to repeat a How without understanding Why is foolish. The popular-end business books don't even worry about that. Their correlation is from three cases based on what the authors want to hear from them. It appears that those authors started with a conclusion, and then cherry-picked the stories that could be fitted to that conclusion. All of the management books are based on the same blue chip companies. Which should make us suspicious anyway because the same companies are used for cases that support competing theories.
The danger of being confused is that we may accidentally slip into chaos. It is because in terms of human perception there is much in common between the Confused and Chaotic domains. Unable to witstand the perception of chaos humans tend to panic and impose too rigid constraints. If the situation is not clear that may lead to a catastrophic fall into chaos. That's why Cynefin's prevents us from jumping into action and instead do a situational assessment first.
Works Cited
1. Kurtz, C. F., & Snowden, D. J. (2003). The new dynamics of strategy: Sense-making in a complex and complicated world. IBM SYSTEMS JOURNAL, 42(3), 22.
2. Snowden, D. (2019, April 19). Liminal Cynefin & “control.” Cognitive Edge. /blog/liminal-cynefin-control/
3. Snowden, D. (2005). Complex Acts of Knowing: Paradox and Descriptive Self-Awareness. Bulletin of the American Society for Information Science and Technology, 29(4), 23–28. https://doi.org/10.1002/bult.284
4. Snowden, D. (2015, July 1). The birth of constraints to define Cynefin. Cognitive Edge. /blog/the-birth-of-constraints-to-define-cynefin/
5. Snowden, D. (2011). Safe-to-Fail Probes—Cognitive Edge. http://cognitive-edge.com/methods/safe-to-fail-probes/
6. Boulton, J. G., Allen, P. M., & Bowman, C. (2015). Embracing Complexity: Strategic Perspectives for an Age of Turbulence (1 edition). Oxford University Press.
7. Snowden, D. (2000). Chapter 12—The Social Ecology of Knowledge Management. In C. Despres & D. Chauvel (Eds.), Knowledge Horizons (pp. 237–265). Butterworth-Heinemann. https://doi.org/10.1016/B978-0-7506-7247-4.50015-X
8. Snowden, D. (2014). Managing the situated present—Cognitive Edge. http://cognitive-edge.com/blog/managing-the-situited-present/
9. Gould, S. J., & Vrba, E. S. (1982). Exaptation—A Missing Term in the Science of Form. Paleobiology, 8(1), 4–15. https://doi.org/10.1017/S0094837300004310
10. Snowden, D. (2019, April 16). Cynefin St David's Day 2019 (2 of 5). Cognitive Edge. /blog/cynefin-st-davids-day-2019-2-of-5/
11. Snowden, D. (2012, December 12). Purpose as virtue: Three elements. Cognitive Edge. /blog/purpose-as-virtue-three-elements/
12. Snowden, D. (2019, April 17). Cynefin St David's Day 2019 (3 of 5). Cognitive Edge. /blog/cynefin-st-davids-day-2019-3-of-5/
13. Snowden, D. (2013, January 21). Granularity. Cognitive Edge. /blog/granularity/
14. McElreath R. (2016). Statistical rethinking: A Bayesian course with examples in R and Stan. Boca Raton, FL: Chapman & Hall/CRC.
Getting started